AI machinas Platform
Automation.
AI machinas started in 2023 with the goal to bring automation powerd by Deep Learning algorithms into masses
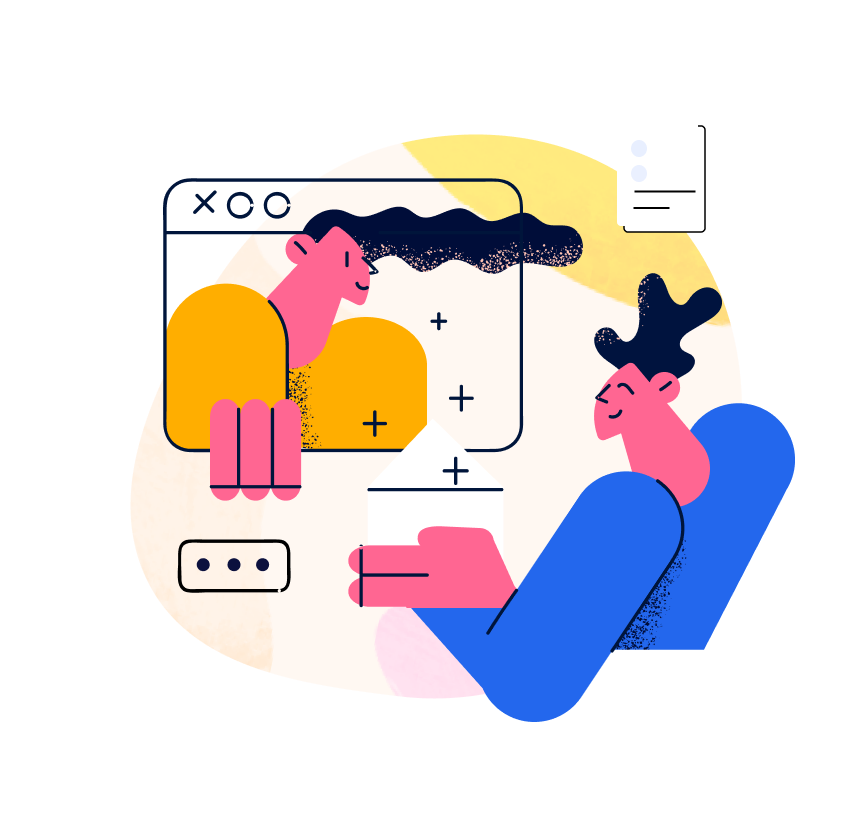
Our Story
AI machinas Automation since 2023!
Deep Learning technology have a huge potential.
We started working on AI algorithms, machine vision long before the idea to build an enterprise grade automation services was born. We realized that AI limitless posibilities can accelerate drastically human achievements by creating the tools that help people to be more creative and faster move forward. Smart working is redefined by AI.
Humble and eager to build something exciting drives us everyday.
0+
Developersuse AI machinas Studio
to bring automation in personal and business projects
0+
Process AutomationDiscovered,
prototyped and built
0k
TasksTemplates
created
Get free AI machinas Studio.
New here? Start your AI automation journey.
Already a member? Sign in.
AI Automation of Business Processes.
AI automation of business processes involves using artificial intelligence (AI) technologies to streamline and optimize various tasks and workflows within an organization. This can lead to increased efficiency, reduced errors, and cost savings. Here are some key aspects and examples of AI automation in business processes: Data Entry and Processing: AI can automate mundane and repetitive data entry tasks, minimizing errors and freeing up human resources for more complex activities. Optical Character Recognition (OCR) technology is commonly used to extract information from documents, invoices, and forms. Customer Service: Chatbots and virtual assistants powered by AI can handle routine customer queries, providing instant responses and freeing up human agents for more complex issues. Natural Language Processing (NLP) enables these systems to understand and respond to user inquiries in a human-like manner. Workflow Automation: AI can be used to automate entire business processes, from lead generation to order fulfillment. This involves creating intelligent workflows that adapt to changing conditions and requirements. Business Process Automation (BPA) tools use AI to optimize and execute complex workflows. Predictive Analytics: AI algorithms analyze historical data to make predictions about future trends and outcomes. This can be applied to demand forecasting, inventory management, and financial planning. Predictive analytics can help businesses make data-driven decisions and avoid potential issues. Document Management: AI can assist in organizing and managing documents by automatically classifying, tagging, and indexing files. Document automation tools use AI to extract relevant information from documents and create structured data. Personalization: AI-driven recommendation engines personalize user experiences in e-commerce, content delivery, and marketing. These systems analyze user behavior to suggest products, content, or services tailored to individual preferences. Employee Onboarding and HR Processes: AI can automate parts of the employee onboarding process by handling paperwork, providing information, and guiding new hires through orientation processes. AI-driven tools can assist in resume screening, candidate matching, and even conduct initial interviews. Cybersecurity: AI is used to enhance cybersecurity by automating threat detection, response, and prevention. Anomaly detection algorithms can identify unusual patterns in network traffic, helping to identify potential security breaches. Robotic Process Automation (RPA): RPA involves using software robots to automate repetitive tasks traditionally performed by humans, such as data extraction and entry. Decision Support Systems: AI systems can analyze large datasets to provide insights and recommendations that assist human decision-making in areas such as finance, marketing, and strategic planning. Implementing AI automation requires careful planning, integration, and consideration of ethical implications. While it can significantly improve efficiency and productivity, organizations should also ensure proper oversight and human involvement in critical decision-making processes.
Robotic Process Automation (RPA) is a technology that uses software robots or "bots" to automate repetitive and rule-based tasks traditionally carried out by humans. These bots interact with applications and systems just as a human user would, by mimicking user actions such as clicks, data entry, and decision-making processes. When combined with artificial intelligence (AI), RPA becomes an even more powerful tool for automating business processes. Here's how AI is often integrated with RPA: Cognitive Automation: AI technologies, such as natural language processing (NLP) and machine learning, are integrated with RPA to enable bots to understand and interpret unstructured data. This can include information from emails, documents, and other sources. Bots can make more informed decisions by learning from patterns and adapting to changes in data. Machine Learning in RPA: RPA systems can be enhanced with machine learning algorithms that allow bots to learn from historical data and improve their performance over time. This enables the automation system to handle tasks that involve decision-making, even in situations where the rules may not be explicitly defined. Advanced Data Extraction: AI-powered RPA can leverage advanced data extraction techniques, including optical character recognition (OCR) and intelligent document processing. Bots can extract relevant information from unstructured documents, such as invoices, contracts, and forms, with greater accuracy. Enhanced Decision-Making: Integrating AI into RPA allows for more sophisticated decision-making capabilities. Bots can analyze data, identify patterns, and make decisions based on predefined rules or machine learning models. This is particularly useful in scenarios where tasks require a level of judgment or adaptation to varying conditions. Adaptive Automation: AI-driven RPA systems can adapt to changing conditions and exceptions. When faced with scenarios outside predefined rules, the system can learn and adjust its behavior, reducing the need for constant manual intervention. Chatbot Integration: RPA can be combined with chatbots, where RPA handles backend processes triggered by user interactions with the chatbot. For example, a chatbot may initiate an RPA bot to update customer information in a backend system. Process Analytics: AI can be applied to analyze the performance of automated processes. This includes monitoring, identifying bottlenecks, and suggesting improvements for optimization. Process mining techniques, combined with AI, can provide valuable insights into how automated processes are functioning. Predictive Analytics: By incorporating predictive analytics, RPA systems can anticipate future events and trends, allowing for proactive decision-making and automation of certain tasks. Integrating AI with RPA can lead to more intelligent, adaptive, and efficient automation solutions. However, it's essential to carefully plan and design these systems, considering factors such as data security, compliance, and the impact on human roles within the organization. Additionally, ongoing monitoring and optimization are crucial to ensure the continued effectiveness of AI-powered RPA implementations.
AI Automation in Insurance.
AI automation is making significant inroads into the insurance industry, transforming various aspects of the business from underwriting and claims processing to customer service and risk management. Here are some ways in which AI automation is being applied in the insurance sector: Underwriting and Risk Assessment: AI algorithms analyze vast amounts of data to assess risk more accurately. This includes data from traditional sources (such as customer profiles and historical claims) as well as alternative data like social media activity and IoT devices. Predictive analytics models help insurers make more informed decisions when underwriting policies, leading to improved risk selection and pricing. Claims Processing: AI automates and expedites the claims processing workflow. Computer vision and image recognition technologies can assess damage from photos, while natural language processing (NLP) is used to understand and extract information from claim documents. Automation reduces the time it takes to settle claims, improves accuracy, and minimizes the potential for fraudulent claims. Customer Service and Chatbots: AI-powered chatbots assist customers in various tasks, such as policy inquiries, claims status updates, and general information. These virtual assistants provide instant responses and enhance the overall customer experience. Natural language understanding allows chatbots to comprehend and respond to customer queries in a conversational manner. Personalized Insurance Products: AI enables insurers to create personalized insurance products based on individual customer profiles and behaviors. This could include usage-based insurance (UBI) for vehicles, health policies tailored to specific health data, and more. Predictive modeling helps insurers anticipate customer needs and offer relevant products. Fraud Detection and Prevention: AI algorithms analyze patterns and anomalies in data to identify potentially fraudulent activities. This includes detecting irregularities in claims, policy applications, and other transactions. Machine learning models improve over time as they learn from historical data and emerging fraud patterns. Operational Efficiency: Robotic Process Automation (RPA) automates routine and rule-based tasks across different departments, such as data entry, policy administration, and compliance checks. AI-driven automation reduces manual efforts, minimizes errors, and enhances overall operational efficiency. Telematics for Auto Insurance: Insurers use telematics data from connected devices in vehicles to assess driver behavior. This data can be used to offer personalized pricing based on actual driving habits. AI algorithms analyze telematics data to assess risk and adjust premiums accordingly. Predictive Analytics for Loss Prevention:
Insurers leverage predictive analytics to anticipate and prevent losses. By analyzing historical data and external factors, AI models can provide insights into potential risks and recommend preventive measures. Customer Insights and Retention: AI helps insurers gain insights into customer behavior, preferences, and satisfaction levels. This information is crucial for tailoring services, improving customer retention, and cross-selling additional products. Compliance and Regulatory Reporting: AI automation assists insurers in staying compliant with regulatory requirements by automating the monitoring and reporting of regulatory changes. This helps ensure that policies and procedures align with current regulations. The adoption of AI automation in insurance not only enhances operational efficiency but also contributes to a more customer-centric and data-driven industry. Insurers need to carefully manage the integration of AI technologies, considering ethical considerations, data privacy, and compliance with industry regulations..
- Tag:
- business,
- makreting,
- tips